What Does the Right-Hand Side of the EDRM Cover?
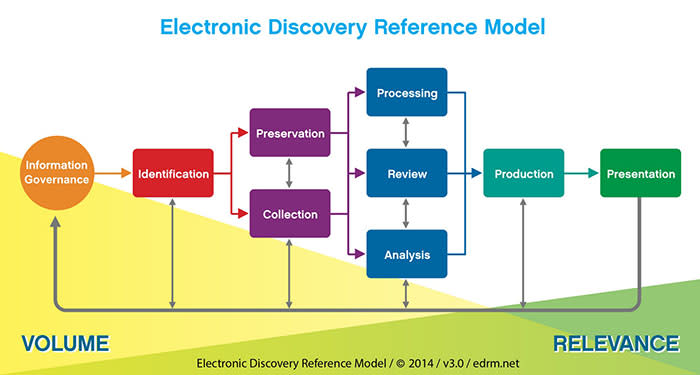
This is the third in our series on our ediscovery chapter of a legal informatics textbook. In this series, we’re covering the ediscovery basics, including the history of the Electronic Discovery Reference Model (EDRM), core technical ediscovery concepts, the technologies powering ediscovery, as well as the future of ediscovery.
Today we’ll walk through our favorite, the right-hand side of the EDRM: data processing, review, analysis, and production. You can also get the ebook in full here.
The “Right-Hand Side” of the EDRM
The right-hand side of the EDRM is primarily the domain of law firms, rather than corporations. Whether it’s outside counsel for a corporation, counsel representing plaintiffs in litigation, or any other representation configuration, it is typically law firms who are charged with performing the tasks on this side. The goal here is to quickly determine what’s relevant to the case and to move those relevant documents forward, whether in a production to opposing counsel, list of deposition exhibits, or any other useful format.
The technology challenges underlying these tasks are inherently more complex, requiring true legal expertise to discover what matters. Software can offer tremendous advantages in that endeavor, in everything from normalizing disparate file types to detecting potential mistakes before they’re committed, but it is ultimately legal professionals who shoulder the burden of marshalling the best evidence in service of the best arguments. In light of that, the best technology in this space augments human expertise, helping those humans reach better conclusions, and faster.
Processing
Processing is a broad term for a set of tasks that ultimately aim to convert collected data into a format suitable for review. It is, in many ways, bringing order to chaos. The input is typically unstructured, collected from a variety of systems, in a dizzying array of formats. The output is clean, normalized, searchable, enhanced data that facilitates rapid and accurate review.
Processing begins with original data as it was collected in its native format, everything from emails to spreadsheets to calendar appointments and meeting notes. The processing steps often include:
Unpacking containers, such as forensic disk images, PST mailbox exports, or ZIP files;
Extracting text, including using optical character recognition (OCR) to recognize text as necessary;
Extracting and normalizing metadata;
Generating images—typically in TIFF format, but increasingly in PDF—to make it possible to view file contents across devices and without native software;
Extracting embedded documents (email attachments, files inserted into Office documents);
Other steps, depending on the processing system and the source data.
Processing tools are as old as ediscovery, and some that are still prevalent today (e.g., LexisNexis LAW PreDiscovery) trace their roots back to those early days. Some are freestanding, flexible applications (e.g., Nuix, LexisNexis LAW PreDiscovery), whereas others are relatively well-integrated into larger suites (e.g., Relativity Processing, Ipro Tech’s eCapture, Everlaw’s processing engine). In all cases, the computing horsepower required is fairly substantial, and throughput is often cited as the chief concern; however, recent advances in cloud-based processing have greatly eased that concern.
Review
This is the heart of the right-hand side of the EDRM—if not all of ediscovery—wherein the data is examined, often document-by-document, to determine whether and how it relates to the matter at hand. Compared to the other phases of the process, this stage has yielded very little to automation, and remains the most labor-intensive part of ediscovery (if not litigation overall). Nevertheless, technology plays a major role in streamlining and enhancing this critical task.
In the context of a party reviewing documents for potential production (e.g., defense counsel, or a government responding to a FOIA request), the primary goals are twofold. First, the producing party seeks to identify data that must be produced because it is responsive to the requests made. Second, the producing party looks to ferret out any data within the set of responsive data that should be withheld because it is privileged or subject to other protections. Most commonly, the producing party attempts to withhold data that are subject to:
The attorney-client privilege, for confidential communications between a person seeking legal advice from a professional legal advisor and that advisor;
The work-product doctrine, for materials prepared in anticipation of litigation or for trial;
Trade secret protection, for confidential information whose value depends upon it remaining secret, and which the owner has taken reasonable measures to protect; and
Privacy law, for information protected by a right to privacy, e.g., financial information about employees and stockholders, or personal financial information.
The process of removing this protected information is called redaction, and redaction may take the form of entirely withheld documents, redacted pages or sections of documents, or specifically redacted words, phrases, or other elements within a given document.
For requesting parties, or those receiving data from producing parties, the perspective is quite different. Rather than responsiveness or privilege, receiving parties are primarily concerned with relevance—and not just relevance in a broad sense, but, typically, relevance to the particular set of facts and issues that form the basis of their complaint. Indeed, the effort here often involves meticulous review and hand-coding to complex classification schemes, all to aid in the downstream preparation of compelling arguments by the litigation team.
On either side, the process is daunting. Timelines and budgets are tight, the volume and variety of data is overwhelming, and mistakes can be incredibly costly. In this environment, every technical advantage helps, large and small. Fast system response times, whether to a search query or a command to load the next document, can shave hundreds of hours off of the cumulative review time. Intuitive interface design can make the difference between continual fumbling and focused progress. And smart defaults and configurable shortcuts can replace halting progress with smooth sailing.
There are hundreds of programs that address this stage of the EDRM, but they have generally evolved over three generations:
The first-generation tools, first taking hold in the 1990s, attempted to move what was previously a largely paper-based process into the digital era. These tools were thus primarily focused on presenting a familiar workflow to practitioners, albeit in electronic form. LexisNexis Concordance is a prime example in this class.
The second-generation tools, appearing in the 2000s, sprang out of a recognition that natively-digital data is inherently different from its paper predecessors. These tools sought to tame the growing wilderness of file types and proliferation of data with more robust backend databases and enterprise-grade user experiences. Relativity is a prime example in this class.
The third-generation tools, established in the 2010s, are primarily products of the cloud. They capitalize on cloud scalability and efficiency to offer the same level of utility—or, in some cases, vastly superior utility—with much more intuitive, consumer-grade user experiences. Everlaw is a prime example in this class.
Analysis
The Analysis task involves breaking the collection down to better understand what it contains and to accelerate the process of review. It is worth noting, though, that this is undoubtedly the most oversimplified part of the framework. Analysis is now a component of nearly every other stage of the EDRM, and the approaches and technologies involved are nearly as varied as the file types feeding the discovery process. To simplify this discussion, we will refer to them more broadly as analytics, focus on the analytics used primarily in review, and divide them into two types: project analytics and document analytics.
Project analytics focus on monitoring, predicting, and improving review performance. Typically, this takes the form of one or more system-generated reports on:
Reviewer pace, the speed at which individual reviewers move through the review process;
Reviewer quality, the accuracy with which individual reviewers classify data in review;
Overall progress and pace, across all reviewers, including estimates for time and cost to the completion of review; and
Live and historical usage data for each reviewer.
Armed with this information, review managers can continually optimize the review process, coaching or replacing underperforming reviewers, securing the necessary time and other resources for successful review completion, and otherwise intervening as necessary in the day-to-day review process.
Document analytics, on the other hand, are focused on delivering greater insight about the nature and contents of the data at hand. The toolkit here is even more varied, ranging from straightforward approaches like email threading, duplicate detection, search term reporting, and coding trend analysis, to more sophisticated techniques, such as:
Data visualization, the graphical representation of aggregate statistical data about sets of documents, to aid in providing an initial understanding of what kinds of documents are in a particular collection;
Entity extraction, the identification of named entities in the data (e.g., people, places, organizations), to aid in the retrieval of information relating to those specific entities, even where they may be referred to in different ways;
Concept searching, the organization of data around key themes in the matter, to improve understanding of the topics covered therein and aid in the retrieval of data related to those specific topics;
Communication mapping, the visualization of communication patterns between people in the matter, to illuminate relationships and anomalous behaviors; and
Predictive coding, using machine learning to teach the system to automatically identify potentially interesting information, to focus limited review resources on the most promising data, avoid the unnecessary review of uninteresting data, and ensure that nothing is misclassified along the way.
These powerful technologies can greatly accelerate the process of review, while simultaneously improving the quality of the output.
While project analytics have been developed more or less organically into existing review platforms, document analytics first rose to prominence as separate applications, offering varying levels of integration into mainstream review platforms. For instance, Equivio and Content Analyst were both market-leading independent companies with proprietary document analytics offerings for ediscovery (Zoom and CAAT, respectively). Their products were offered alongside dozens of review platforms, delivering bolt-on, on-demand analytics to systems otherwise lacking such tools. Within the span of 14 months, however, both were acquired by larger organizations looking to integrate those analytics into their ediscovery suites (Equivio by Microsoft, and Content Analyst by Relativity). Today, these analytics are increasingly viewed as table stakes, and many ediscovery platforms have at least modest capability in this realm.
Production
Production is the act of handing the responsive and non-privileged data over to the other side, ideally in a format that they can easily use for their own review. By this stage, the set of responsive, non-privileged data has been defined, so the challenges here revolve primarily around the efficient and effective packaging of that data for transfer to opposing counsel. Not surprisingly, the standards in this space are constantly evolving, and are subject to some of the most intense negotiation in the ediscovery process.
A standard production is relatively structured, when compared to the unstructured source data from which it derives. It typically contains the following elements:
A set of images for each document (except those not reasonably imaged, like spreadsheets), most commonly in TIFF format, but increasingly as PDFs;
A set of text files containing the text extracted from the source documents or generated with OCR software;
The native source files, at least for those document types for which native files were agreed upon (e.g., spreadsheets); and
A load file, tabular data correlating all of the other files and including any metadata extracted from the native source files, all following an agreed-upon order and labeling convention.
The precise parameters of the production—Should natives be produced? What format for the image files? How should redactions appear? How should the load file be formatted? What metadata should be produced?—are intensely negotiated and recorded in the form of a production protocol. Over the years, litigants looking to simplify this process have settled on several protocols or protocol components that have become de facto industry standards (e.g., a Concordance-style load file). Government bodies with significant litigation loads and leverage have also established their own standards (e.g., the SEC has their own standard production protocol for data they request).
All of the data to be produced is typically packaged into an encrypted file and/or onto physical media, and delivered to opposing counsel as circumstances and/or mutual agreement dictate (e.g., via Secure File Transfer Protocol, cloud file repository, courier, etc.). The receiving party then ingests the packaged data into their ediscovery platform—hopefully without issue, if they successfully negotiated the production protocol—and begins their own review of the data.
Most of the work here revolves around the transformation of data, so it is not surprising that many of the same tools used in the Processing stage are also used in the Production stage. As with processing, one has a choice between freestanding applications (e.g., LexisNexis LAW PreDiscovery) or those built into review platforms (e.g., Relativity’s production sets, Ipro Eclipse productions, Everlaw’s production tools). And throughput is also the major challenge, but recent advances in cloud-based production tools have alleviated that problem.
Presentation
Presentation is the curation and use of the most relevant data to help make your case, both at trial and in the steps leading up to it (e.g., hearings, depositions, motion practice, etc.). It’s worth noting that, from an EDRM standpoint, this is a fairly broad definition. Given their charter, the EDRM drafters were more concerned with the actual rendering of exhibits in the real world than with fact development or case strategy. The latter is what bridges the gap, however, between the process of finding relevant data and the act of presenting them as evidence before a judge or jury.
The approaches to developing a case are as varied as the cases themselves, but there are nonetheless several common techniques that benefit greatly from modern data management technology. They include:
Assembling a database of facts, usually with extensive cross references to the relevant people, places, organizations, and events in the case, and with annotations as to which facts favor which party, which are contested, and which remain unsupported by evidence.
Building a case timeline, or chronology, to understand the temporal order of the data—through visualization, preferably—and to illuminate gaps, patterns, and hot spots.
Creating an outline of the key points to make in a deposition, hearing, or motion, also with extensive cross-references to the entities involved and the relevant data discovered during review.
Reviewing and organizing the output from depositions—including audio, video, transcripts, and exhibits—with an emphasis on incorporating newly-discovered information into the overall case strategy.
The tools at this far end of the EDRM are sometimes not connected to ediscovery from a technology or marketing perspective. Rather, they are positioned as “case management” tools and are designed to ingest data from various sources, including ediscovery software (e.g., Thomson Reuters’ Case Notebook). Some software is integrated, to varying degrees, into a suite of tools that ultimately cover most of the EDRM (e.g., LexisNexis’ CaseMap case management and Sanction trial presentation software, which offer some integration with their Concordance review software). Finally, some ediscovery systems offer this functionality as part of one tightly-integrated package (e.g., Everlaw’s StoryBuilder features, an inseparable part of the SaaS ediscovery platform).
Stay tuned next time when we dive into the technologies powering modern ediscovery, beginning with cloud computing.