What Is Predictive Coding, and How Does It Apply to Ediscovery?
by Giulianno Lopez
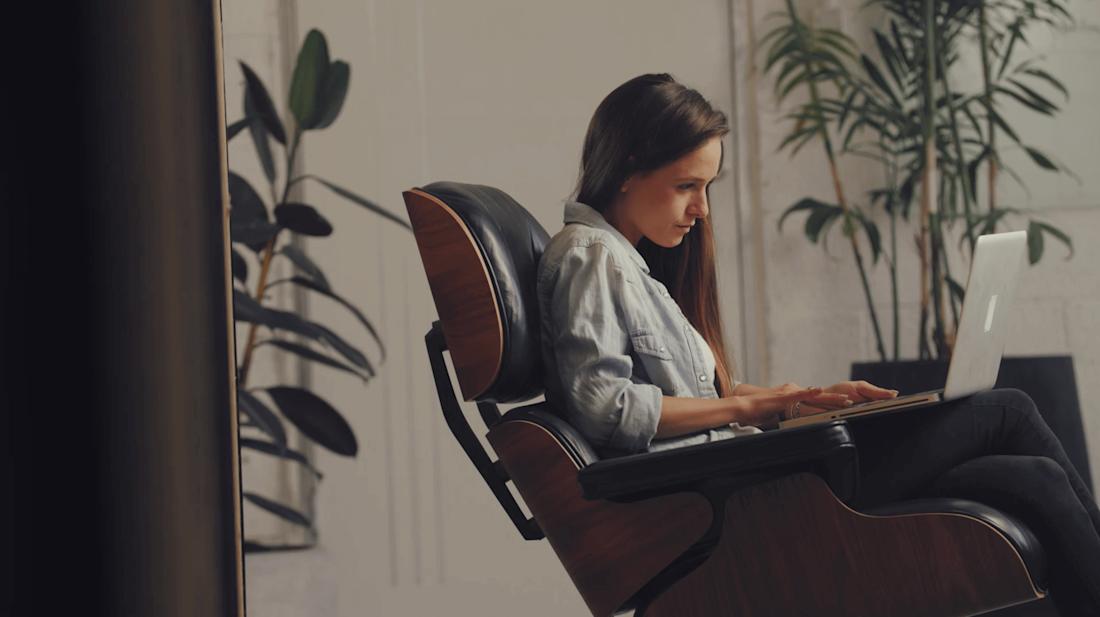
Before ediscovery tools became a common part of litigation, legal teams had no choice but to manually review every document requested and provided during the discovery process. However, manual review became impractical when modern data formats and technology made the volume of discoverable information explode. Predictive coding is now transforming ediscovery, using artificial intelligence (AI) to expedite the review process, saving firms both time and money.
What Is Predictive Coding?
Predictive coding leverages machine learning to surface likely relevant documents based off prior review decisions. Although machine learning may sound mysterious, it is actually a part of everyday life for most people. Consider the following examples:
Netflix and Amazon give movie and television recommendations based upon prior viewing choices.
Google search results are based on an algorithm that learns from past search and internet browsing behavior.
Spam filters allow us to divert dozens of unwanted emails from our inboxes.
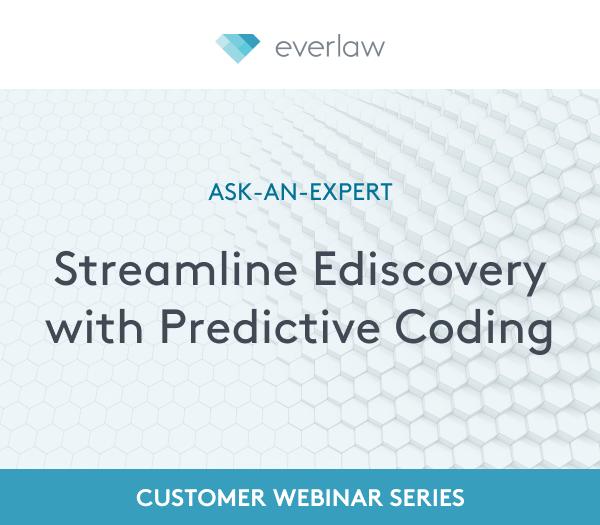
Tune in to our CLE-accredited webinar as we break down predictive coding, review industry standards and related court rulings, and highlight the best opportunities to leverage predictive coding. The live webinar session is expected to qualify for up to 1.0 hour of CLE credit. Viewing this session on-demand will not qualify for CLE credit.
The Role of Predictive Coding (and Human Reviewers) in Ediscovery
Within the realm of ediscovery, predictive coding — also known as technology-assisted review (TAR) — automates document review. A type of machine-learning technology, predictive coding gives computers the ability to learn from human input and make educated guesses when classifying documents. Legal professionals widely use predictive coding to identify responsive or relevant documents and data in other review categories, including privilege, issue codes, and other vital classifications, in order to dramatically reduce document sets — sometimes from several million down to a few thousand.
Legal professionals can leverage predictive coding technology to find responsive electronically stored information (ESI) during the review phase of a case, shaping and altering the discovery process for law firms, lawyers, clients, and the courts. Here’s how it works:
The software is “trained” with a seed set of data — a sample of documents retrieved from the larger group of documents needing review.
Reviewers code each document as relevant or not relevant to the case and input this information into the predictive coding software.
As document review continues, artificial intelligence enables the software to continually learn from reviewer decisions and make faster and more accurate decisions.
The predictions generated by predictive coding can be extremely powerful. They can uncover a “smoking gun” document that will serve as proof in a criminal case, avoid an extensive manual review of documents to save thousands or even millions of dollars in review time, prioritize documents for relevance review to make the process increasingly efficient, and be used for quality control, making the review process significantly more accurate. Given these benefits, it is not surprising that a majority of corporate counsels are using predictive coding in their cases, and courts are routinely approving the use of the technology in the cases before them.
Predictive Coding in the Everlaw System
Predictive coding with Everlaw learns continuously from reviewer decisions, enabling reviewers to use their standard workflow — assigning ratings, codes, and attributes to reviewed documents — to teach the system how to find more relevant documents on their behalf. Everlaw users can review up to 86 docs/hour with standard review and 140 docs/hour with automatic context coding and search and review data, including spreadsheets, PDFs, CAD files, videos, mobile data, medical images, and modern chat programs.
For more information on how predictive coding works and how it works within the Everlaw platform, check out our CLE-accredited webinar, “Ask an Expert: Join our Predictive Coding Webinar.”
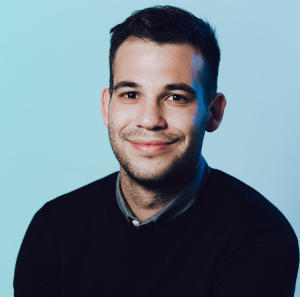
Giulianno Lopez is a content marketing manager and screenwriter who is passionate about research, writing, and data.
Embrace the power of automation
Predictive coding is just one of the tools lawyers need to be successful in the digital age. See how Everlaw is creating the features future attorneys want.
Request a Demo
Thank you
We’ll be in touch soon.
Can’t wait? Feel free to call us at 1 (844) EVERLAW (383-7529).