Automating The Manual Components of Ediscovery (Part 2): Reviewing Modern Data
The unprecedented increase of electronically-stored data in modern litigation and internal investigations continues to complicate things for the legal community. This uptick in volume makes it even more challenging for legal professionals to scour hoards of data and find relevant information — all under tight deadlines. Modern data sets can contain file types that are challenging to redact, and their larger file sizes require more reviewers, which also increases the probability of human error.
Yet many legal organizations find it’s not feasible to rely on outside certified administrators, managed review vendors, or sophisticated in-house ediscovery platforms (that often charge hefty project management fees to meet their deadlines) when trying to keep costs low for clients. However, ediscovery automation technology can help accelerate legal work, create more efficient workflows, and ensure consistency when reviewing, coding, and redacting data.
Ediscovery Pain Points When Reviewing Modern Data
As data volumes have exploded, so has the prevalence of similar or duplicate documents among data sets. This hiccup can amplify the potential for inconsistent coding decisions among different legal teams, especially when reviewers have to manually update similar documents one at a time. Ultimately, reconciling coding differences among reviewers slows down the review process and increases costs.
Redacting large spreadsheets can also further slow down review timelines. Traditionally, legal teams convert spreadsheet files into images in order to create lasting and complete redactions. This would often make the formatting on large, complex files unrecognizable. As a result, reviewers would have to spend hours drawing black boxes across identical content spread across many pages with little reference to its place in the original spreadsheet.
Critical evidence in litigation or investigation can often come from audio and video files. It’s often necessary to transcribe A/V files in order to create an organizable, searchable written record of their contents. Traditionally, this means a costly manual transcription of their contents, which can also further delay project timelines.
Streamlining Review With Automation Technology
Ediscovery platforms such as Everlaw can address the pain points that arise when reviewing modern data files. Everlaw allows legal professionals to easily upload documents to the platform and review and redact various data file types. These capabilities create a more efficient workflow and eliminate wasted time spent completing manual and time-consuming tasks.
Auto Code Contexts
Auto-code contexts are an essential component of ediscovery platforms as they provide project administrators precise controls, within a given case, to enforce consistency in the review process. Ultimately, helping drive consistency, streamline the review process, and increase confidence in the quality of coding decisions. This feature allows a project administrator to set rules to apply consistent coding across all documents within a specific context.
For example, Everlaw’s auto-code contexts enable project administrators to set rules for duplicates, attachments, or email threads. This feature eliminates unnecessary work for reviewers when they make coding decisions since contextually-related or duplicated documents are automatically coded.
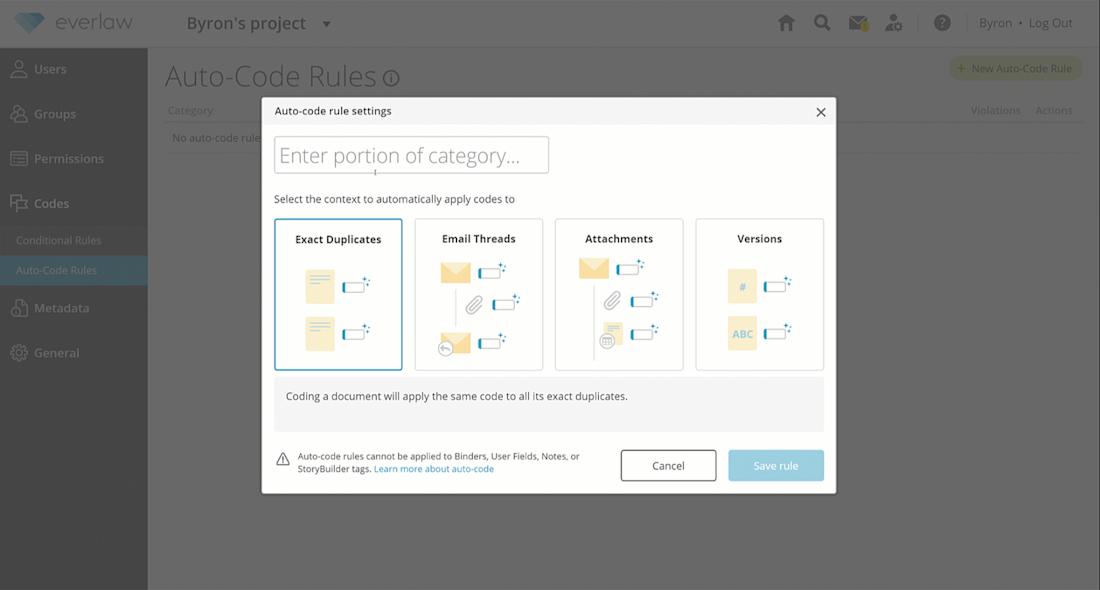
Native Spreadsheet Redactions
There are very few things as tedious as manually redacting the same information over and over again. Some ediscovery platforms solve this pain point by enabling legal professionals to redact the same term across multiple documents via batch actions. This removes repetitive steps and improves accuracy by applying the same review decisions to all documents within a specific context.
For example, Everlaw eliminates the drudgery of converting large spreadsheet files into images and then manually redacting cells by enabling users to make redactions directly within native spreadsheet files. Redacting the same term across many documents is as easy as selecting the documents in question, identifying the words, phrases, or patterns in question (such as PII, PHI, PCI, etc.), and applying that selection. As a result, users can complete reviews with ease and flexibility without the need to manually redact each document.
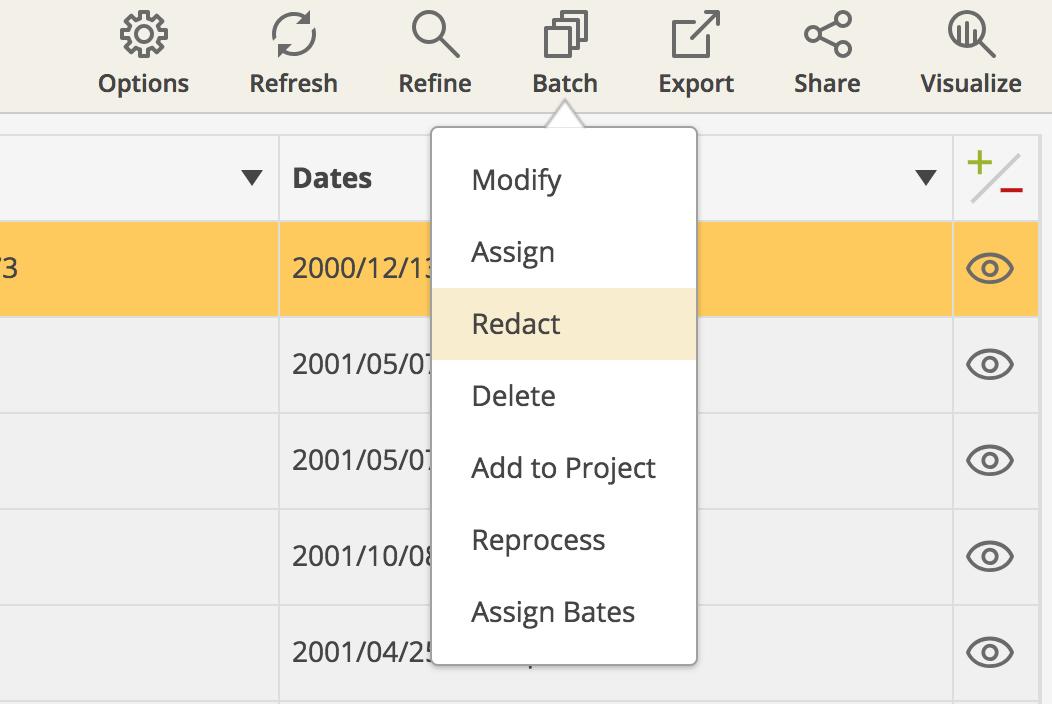
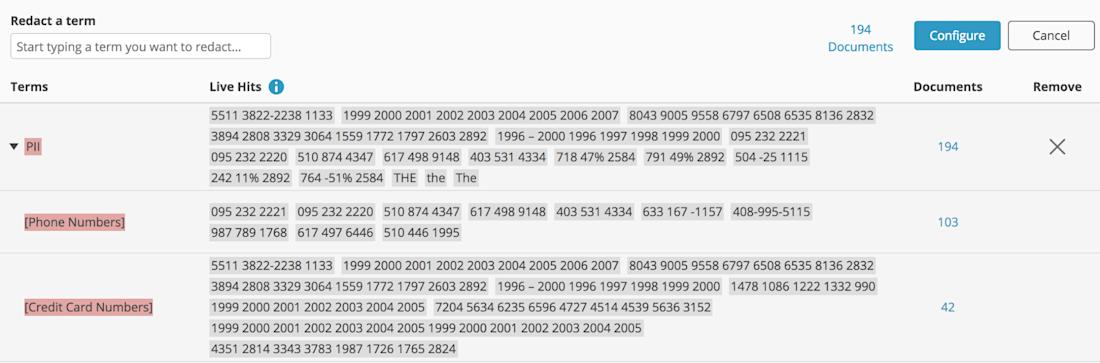
A/V Transcription
Reviewing and transcribing audio and video evidence can often be a time-consuming ordeal. Fully automated A/V transcription capabilities help alleviate this by creating searchable transcripts of audio and video files. Additionally, timestamped, searchable notes can be added during the review process.
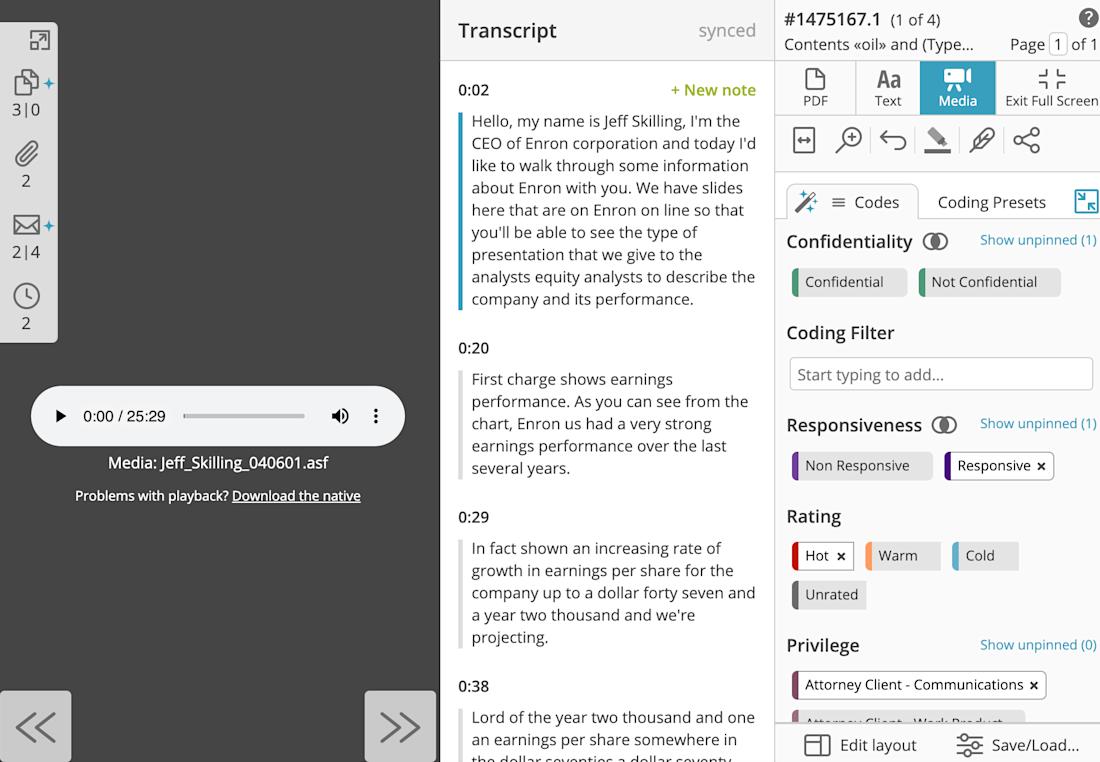
For example, Everlaw enables reviewers to utilize search against the text of A/V files and incorporate that text into predictive coding models. This significantly reduces the cost of transcription services and provides an instant window into previously opaque information.
Predictive Coding
Predictive coding continues to transform ediscovery, reducing the amount of data that needs to be reviewed by upwards of 80% in some matters. When predictive coding technology operates through continuous active learning as it does on ediscovery platforms, it eliminates the need for explicit human input via training sets and automates the process of machine learning.
For example, Everlaw’s predictive coding technology continuously learns from reviewer decisions, enabling reviewers to use their standard workflow — assigning ratings, codes, and attributes to reviewed documents — to teach the system how to find more relevant documents on their behalf. This enables both novices and power users to easily create a predictive model via the wizard-driven process.
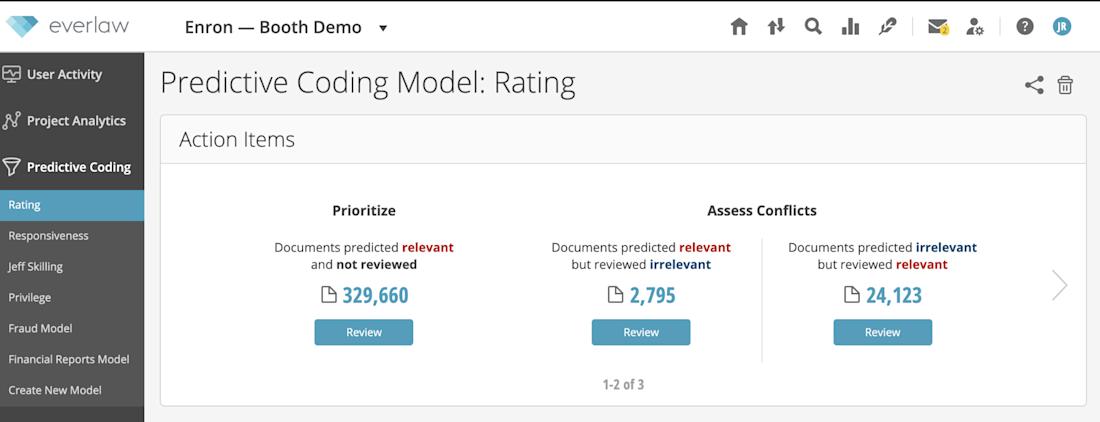
The ease of creating a model ensures that a variety of use cases can leverage predictive coding to enhance efficiency. These use cases include surfacing the most relevant predictions or helping to ensure quality control in a collaborative document review.
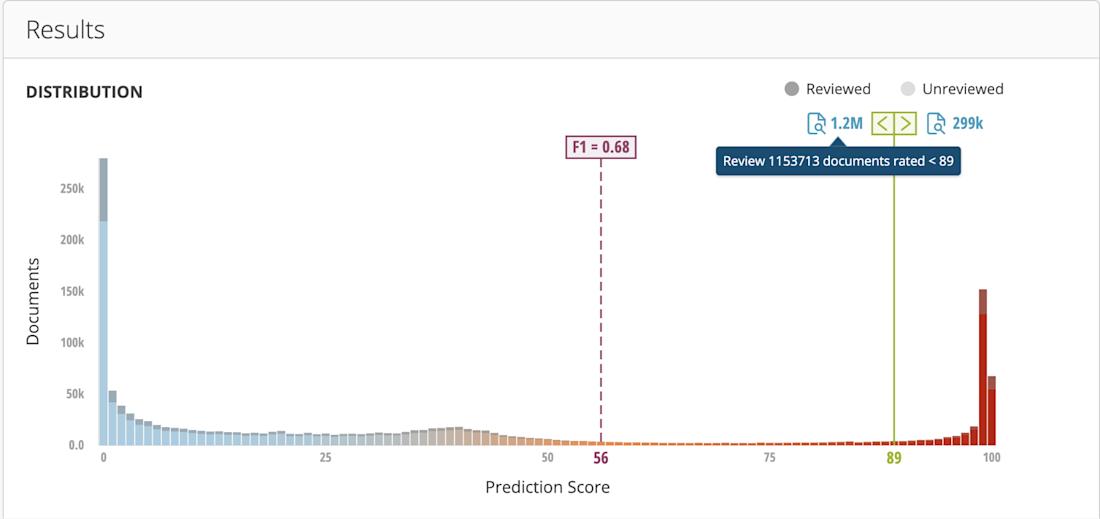
The creation of a predictive coding model uses the same visual, color-coded logical operators and search interface seen elsewhere on the platform. This makes it easy to benefit from the power of artificial intelligence without paying more for the functionality or relying on a certified expert to build and manage the models.
Moving Forward
When project timelines are tight, and data volumes are massive, saving time on manual tasks helps law firms and corporations achieve better outcomes. In part three of this blog series, we will explore how automation helps legal teams collaborate efficiently on productions and when preparing to present the facts they’ve uncovered.
For more information on how Everlaw customers have been using our ediscovery automation technology in their legal work, check out our whitepaper on Ediscovery Trends and User Insights: A Year in Review