Predictive Coding in the World of Ediscovery
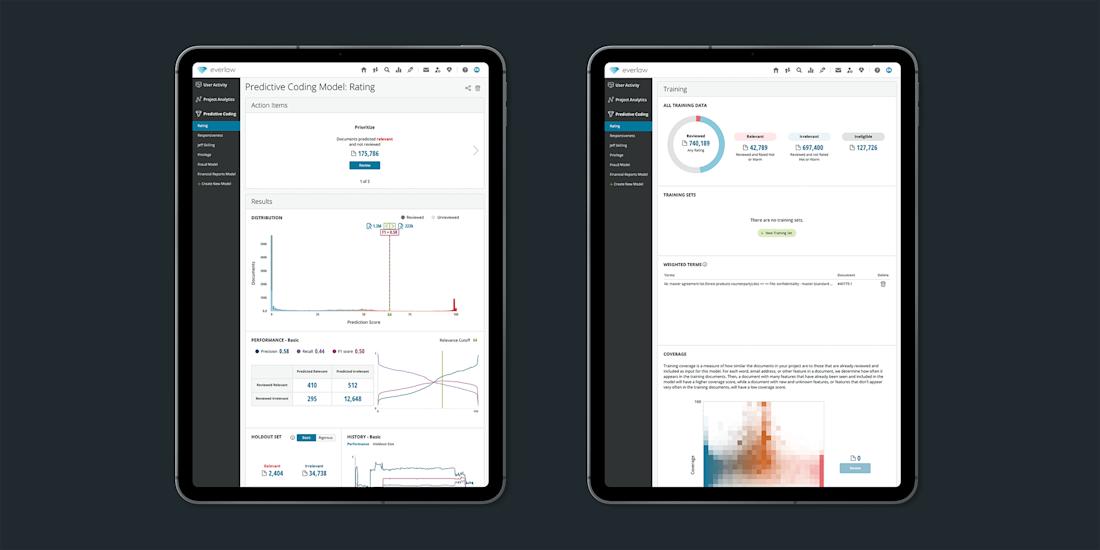
The human brain is continually being fed heaps of sensory information that must be processed and acted upon quickly. One way to significantly improve this process is to predict incoming information based upon previous experience. The projected information can be processed efficiently, and anything unexpected can be dealt with accordingly. This is the concept behind predictive coding.
The Connection Between Predictive Coding, Artificial Intelligence, and Ediscovery
In the context of ediscovery, predictive coding is a type of computer-assisted review (CAR), also known as Technology-Assisted Review (TAR), that uses artificial intelligence to categorize documents based on a sample set of documents. This process can dramatically drill down the number of documents in extensive collections to include only those relevant to a specific matter.
Artificial intelligence (AI) gives a computer the ability to perform tasks that were previously completed by humans. Predictive coding uses AI to automate these manual tasks, an increasingly valuable function, considering the massive amount of data currently being generated during the ediscovery process. When powered by AI, ediscovery becomes a more frictionless process — allowing legal teams to get a handle on the facts of a case with greater speed, efficiency, and accuracy at a much lower cost.
The number of electronic documents that must be reviewed in ediscovery has grown dramatically over the last decade. With predictive coding, rather than reviewing every document within a collection, a reviewer can achieve similar results after reviewing only a relative few. Essentially, predictive coding expedites the review process by leveraging artificial intelligence (AI) to surface relevant documents based on previous review decisions.
Sound like magic? Not quite.
Even though predictive coding is a highly effective way to cull data sets to save time, money, and effort and helps with review prioritization and quality control, it does not replace all human review. Instead, it projects the likelihood of relevance within a collection based on how it has been trained to date. This allows for one or several people to efficiently review millions of documents in a relatively short period of time, with higher accuracy and consistency at a lower cost than with traditional review methods.
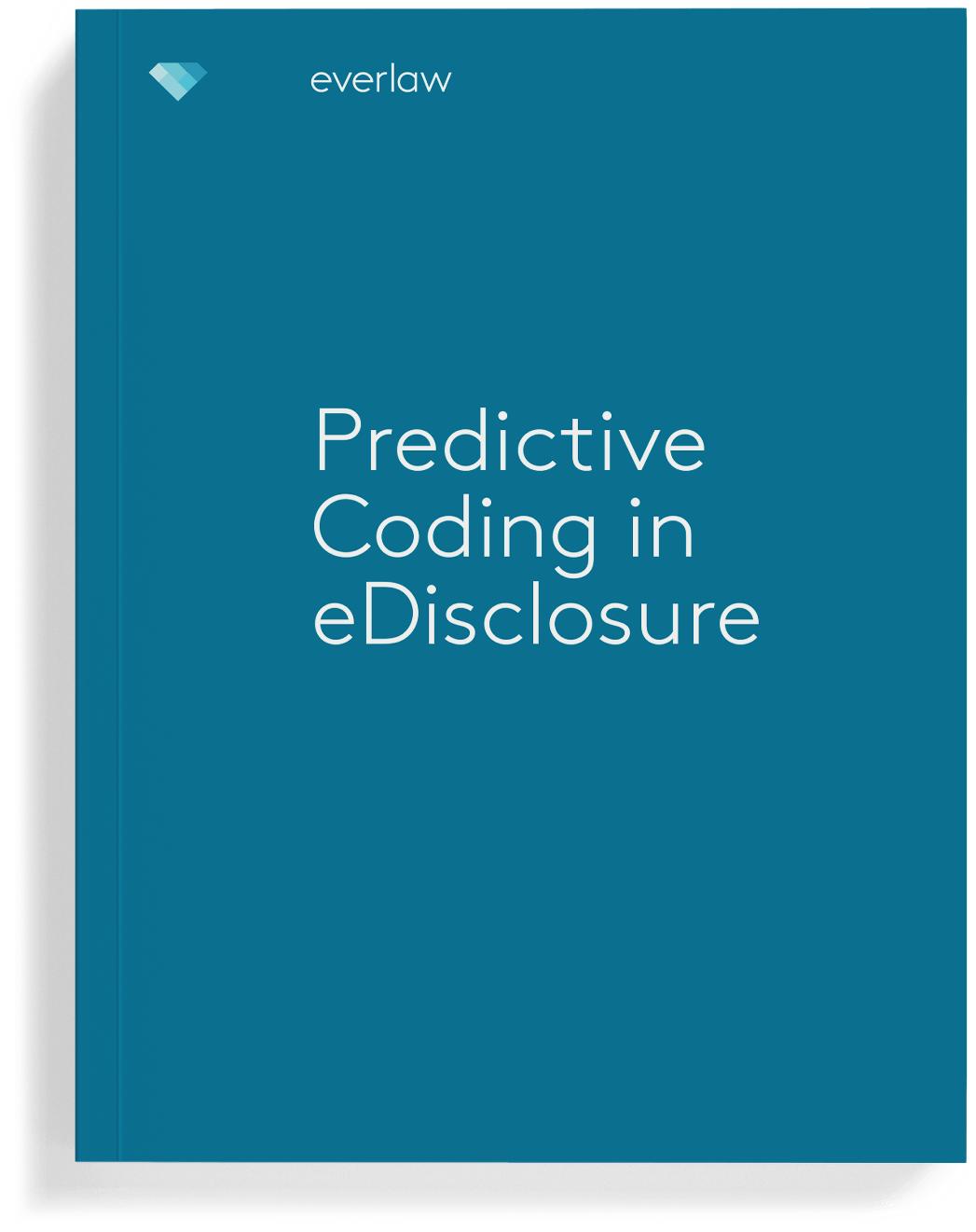
How Predictive Coding Works
The premise behind predictive coding is to locate documents comparable to those that have been determined relevant or not relevant by a person of authority. It’s based on binary rating systems that help to identify and classify documents based on their relevance. However, the computer does not impose its own judgments about the responsiveness of documents; instead, it seeks to match the decisions made by an authoritative source.
Predictive coding utilizes numerous technologies to formulate its decisions, including:
Latent semantic analysis and probabilistic latent semantic analysis. This summarizes the meaning of words by comparing documents containing those words.
Support-vector machine. This attempts to find a line separating responsive from non-responsive documents.
Nearest neighbor classifier. This categorizes documents by locating an already-classified example that is very similar to the document being reviewed.
Active learning. This presents reviewers with documents most likely to be misclassified.
Language modeling. This summarizes the meaning of words based upon how they are used in a set of documents.
Relevance feedback. This adjusts the criteria for indirectly identifying responsive documents based on input from a knowledgeable user.
Linguistic analysis. This attempts to maximize the correct classification of documents through semantics.
Naïve Bayesian classifier. This scrutinizes the probability that each word in a new document originated from the word distribution of trained responsive or non-responsive documents.
While most of these systems involve machine learning, their accuracy depends on the specifics of the implementation and the quality of the training set used.
Applying Predictive Coding
Although predictive coding may sound complex, its application is relatively simple. One of the most important aspects is having an authoritative reviewer or team of reviewers with sound judgment and a high level of expertise. The categorization of potentially millions of documents depends upon their determinations regarding which documents should be labeled relevant and which shouldn’t be.
It’s important to note that predictive coding software will follow correct and incorrect guidance, whatever the trainer feeds it. Suppose an outside contract attorney, with little expertise in the subject matter of a case, codes a large number of relevant documents as not relevant. In this example, the systems will then conclude that other potentially relevant documents in the data set may not be relevant because of how the attorney trained the model (even though they may very well be).
There are several ways to train a predictive coding system:
Provide a “seed set” of pre-classified documents on which to base the training.
Randomly choose documents and provide them to an expert for classification.
Combine a pre-planned set with random sampling.
When trained and used correctly, predictive coding can be a powerful tool when performing ediscovery. Not only can predictive coding significantly reduce the sheer volume of documents that must be produced, but it can also lead to greater levels of consistency, usefulness, efficiency, and accuracy.
The producing party will generate more focused documents more cost-effectively, and the requesting party will obtain a more complete production of documents in a shorter amount of time. Maybe not magic, but a definite win-win for legal professionals and consumers.
What This Cutting-Edge Technology Means for Everlaw Users
Everlaw has the fastest review speeds (from 86 docs/hour with standard review to 140 docs/hour with automatic context coding) combined with accurate, AI-powered search. Users have the ability to search and review data from PDFs, spreadsheets, videos, CAD files, medical images, mobile data, and modern chat programs — all within the same platform.
For example, McDonald Carano, Nevada’s premier law firm, utilized Everlaw’s combination of instant search, visual email threading, and date filters to decrease the initial review corpus by 76%.
With Everlaw, the firm used Everlaw’s Predictive Coding to surface the most relevant documents (based on their previous coding decisions), helping to reduce the initial data volume by 96%. After identifying and pooling all the relevant documents, Everlaw’s production wizard enabled the team to produce the relevant documents quickly and share the results.
Everlaw handles email conversations better than any other platform, displaying email threads in a visually intuitive way that puts everything in context and is easy for attorneys to understand.
To find out more about how predictive coding and artificial intelligence work within the Everlaw system, download our white paper, “Tactical Review with Predictive Coding,” today.