Ediscovery Automation: Streamlining Mid-Project Tasks with Everlaw
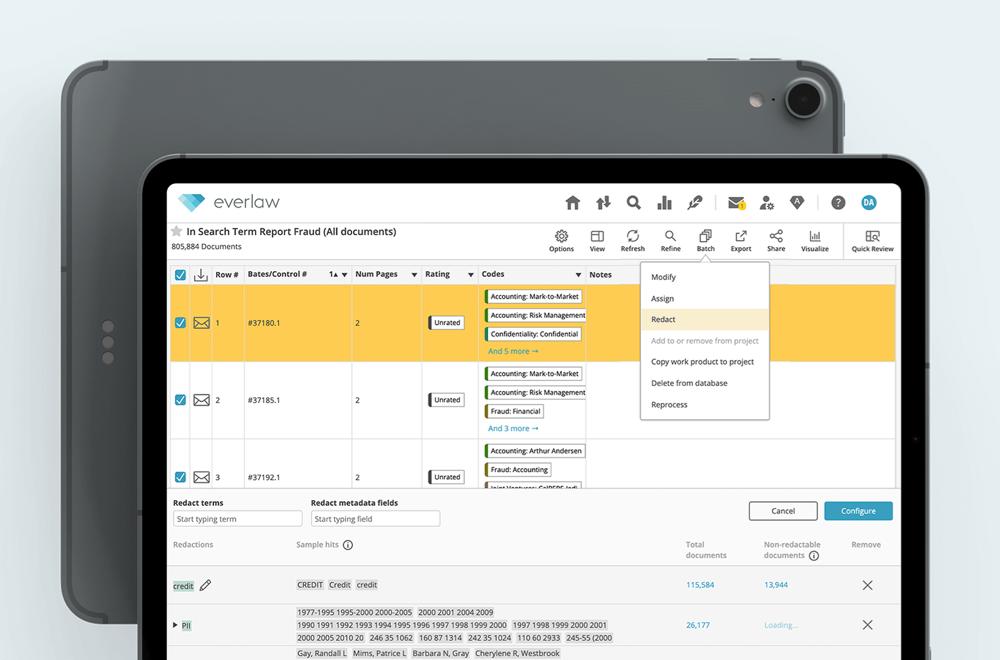
During the discovery stage, legal professionals are often burdened with numerous tedious tasks that slow down an already arduous process. Ediscovery automation helps streamline workflows by reducing the likelihood of human error, which causes delays, increases costs, and lowers the quality of legal work.
Everlaw’s ediscovery automation technology eliminates many tasks that would otherwise be painstaking manual processes on other platforms. In particular, the processes of coding and redacting identical information across similar documents, redacting native spreadsheet files, audio and video (A/V) transcription, and creating training sets for technology-assisted review are fully automated on the Everlaw platform.
Auto-Code Rules
Consistent ratings and coding decisions between related documents are essential to ensure an accurate and uniform review process. Having to remember to manually update all related and duplicative documents, one at a time, is highly likely to result in human error. Batch updating is a partial solution but still doesn’t altogether remove the possibility of inconsistencies.
When this is compounded across a large team of reviewers on a project, the inconsistencies can rapidly pile up. This creates more work for admins to reconcile coding differences, ultimately slowing down the review process and increasing costs.
Auto-code contexts on the Everlaw platform provide project admins precise controls within a given case to enforce consistency in the review process. This feature allows project admins to set rules to apply consistent coding across all documents within a specific context.
For example, project admins can set rules for duplicates, attachments, or email threads so that as a reviewer makes a coding decision for one document, contextually related or duplicative documents will be coded automatically. This drives consistency, streamlines the review process, and increases confidence in the quality of coding decisions.
Additionally, auto-code contexts help expedite the review process. With auto-code rules set up accordingly, less time is spent performing quality control. When conducting privilege review, admins can set up auto-code rules to code documents as privileged or confidential if any exact duplicates are coded as such.
In the "Automating Ediscovery from Start to Finish with Everlaw" white paper, we dive into how Everlaw’s ediscovery automation technology streamlines the ediscovery process, from data upload and processing to search, review, and production.
Redactions
Redactions are a fundamental part of responsible and secure litigation, not to mention that they can make or break the credibility built with a client. Gone are the days of grabbing a black Sharpie and crossing out personally identifiable information (PII) or attorney-client privileged information from paper documents. Applying redactions to conceal confidential or privileged information is standard procedure during any ediscovery review.
Redacting Spreadsheets
In many modern document bodies, critical information is in spreadsheets. It’s vital that the producing party redact any privileged information before turning the documents over to opposing counsel. These spreadsheets can range from simple documents to files with multiple sheets and thousands of columns and rows.
Traditionally, to create a lasting and complete redaction of this information, spreadsheet files were typically converted into an image form. This would often make the formatting on large, complex files unrecognizable, and reviewers would have to spend hours drawing black boxes across identical content spread across many pages with little reference to its place in the original spreadsheet. Redacting these image files used to be highly inefficient.
Learn how Everlaw’s cloud-based platform and ediscovery automation technology facilitates secure digital redactions.
Batch Redactions
Redactions have always been a tedious process, requiring legal professionals to go through each individual page. Batch redactions solve this by enabling search and redaction of words and phrases for multiple documents all at once — without needing any eyes on review. This is especially important when working with a large data set.
Everlaw eliminates the manual drudgery of converting large spreadsheet files into images and then manually redacting cells by allowing our users to make redactions directly within native spreadsheet files. Redacting the same term across many documents is as easy as selecting the documents in question and identifying the words, phrases, or patterns in question (e.g., PII, PHI, PCI, and so on) and applying that selection.
Everlaw users can complete review with ease and flexibility without manually redacting each document, eliminating repetitive steps and improving accuracy by applying the same review decisions to all documents within a specific context. Users can set up automatic searches for numerous types of PII, including, but not limited to, bank account numbers, passport numbers, employer identification numbers, Social Security numbers, and phone numbers.
A/V Transcription
Audio and video files can be a critical source of evidence in litigation as well as internal investigations. It’s often necessary to transcribe A/V files to create a written record of their contents, making them easy to organize and search. However, manual transcription can be costly and time-consuming, creating cost overruns and delays in ediscovery.
A/V transcription on the Everlaw platform is fully automatic. Upon uploading A/V files, Everlaw creates fully searchable transcripts that can be viewed alongside the built-in media player. Additionally, time-stamped, searchable notes can be added during review. This enables reviewers to utilize search against the text of A/V files and incorporate that text into predictive coding models, significantly reducing the cost of transcription services and providing an instant window into previously opaque information.
Video conferencing tools are creating discoverable data, and as a result, handling A/V files during the discovery stage is causing ediscovery issues. Learn how our ediscovery automation technology is helping to address these challenges.
Predictive Coding
The unprecedented increase in the volume of electronically stored information involved in modern litigation and internal investigations has not lessened the pressure to find relevant information under tight deadlines. Predictive coding has emerged as a partial solution to the problem. So, what exactly is it?
Predictive coding uses artificial intelligence (AI) to extrapolate relevant decisions from human review. Effectively, you identify the kind of documents you want to find more of, and it shows you where in your haystack you should look to find them. It has completely transformed ediscovery, reducing the amount of data that needs to be reviewed by upward of 80% in some matters. However, not all predictive coding solutions are equally efficient.
In ediscovery, this machine learning approach is deployed in predictive coding systems — also known as technology-assisted review, or TAR — that generally fall into one of two categories:
Simple passive learning (or TAR 1.0):
A subject matter expert classifies some documents for training, which the system then uses to test the predictions’ reliability as more documents are classified. Once the performance is acceptable, the prediction model is rolled out to all remaining documents.
Continuous active learning (or TAR 2.0):
Review decisions automatically train the system, and the system continually updates the predictions as new human classifications are made.
TAR 2.0 has many advantages over TAR 1.0, which requires experts to do the initial training and is less effective because it can’t learn from subsequent decisions. TAR 1.0 also cannot handle rolling productions without having to start over. Finally, TAR 1.0 doesn’t work well when the proportion of relevant documents is low.
As a TAR 2.0–based system, predictive coding on Everlaw vastly reduces the need for explicit human input via training sets and automates the process of updating and improving machine learning. Everlaw predictive coding technology continuously learns from reviewer decisions, enabling reviewers to use their standard workflow — assigning ratings, codes, and attributes to reviewed documents — to teach the system how to find more relevant documents on their behalf.
Predictive coding allows legal teams to focus their time on the core details of a case; however, what is often unclear is how it works within ediscovery. Learn more in this post.
This allows both novices and power users to easily create a predictive model via the wizard-driven process. The fact that Everlaw has a wizard-driven process is novel in and of itself. Anyone can create a model, not just a trained tech. For example, an attorney can use it to find more docs for a deposition happening for a specific week. The ease of creating a model ensures that the powerful capabilities of predictive coding can be applied to a variety of use cases, including surfacing the most likely to be relevant documents or helping to enable quality control in a collaborative document review.
The creation of a predictive coding model uses the same visual, color-coded logical operators and search interface seen elsewhere on the platform. This makes it easy to benefit from the power of AI without paying more for the functionality or relying on an expert to build and manage the models. That being said, users can also set up a predictive coding model around the review decisions of a subject matter expert/small group of experts at their discretion.
Are you interested in learning more about how to leverage ediscovery automation technology for your project tasks? Download your free copy of our latest white paper, “Automating Ediscovery from Start to Finish with Everlaw.”