Predictive Coding Best Practices
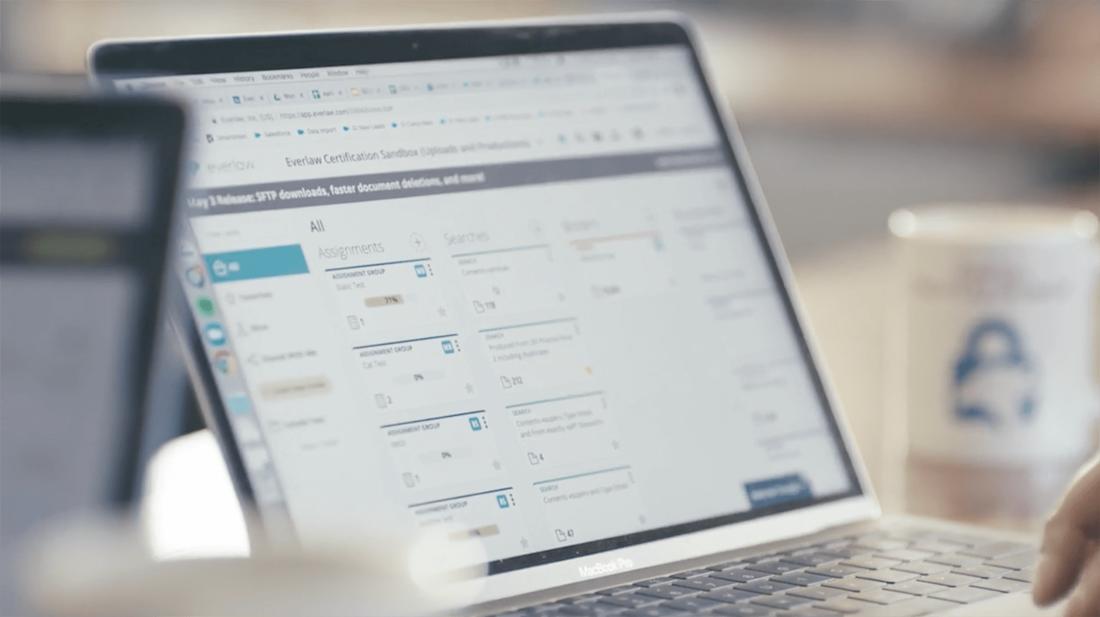
Suggesting how to improve a case’s predictive coding model can be difficult. This isn’t because of any characteristics of machine learning. Rather, providing predictive coding best practices can be tough because of the nature of litigation. For example:
Specific targets for precision, recall, or F1 scores may be set during negotiations with opposing counsel, thereby limiting the flexibility a team has to optimize its model.
Teams may have existing workflows that determine target values for these metrics. For example, one review team might want their predicted scores to be highly accurate and be willing to sacrifice recall. Another review team might want their model to capture as many potentially-relevant documents across a case and sacrifice precision to find them.
Different case types may benefit from different approaches, making it difficult to provide set standards for precision, recall, and F1 that would apply in all situations. Without knowing the case dynamics and unique elements, optimization becomes more difficult.
Despite these possible limitations, there are still ways you can improve your predictive coding model’s performance – and your case outcomes. Here are a few such best practices:
Avoid rating and coding inconsistencies
You’ve likely heard the expression, “Garbage in, garbage out.” It underscores that consistent, meaningful data must inform a model in order for the model’s results to be accurate and useful. Most of us agree with this conceptually, but we struggle to implement it.
The most effective way to get clean data is to prepare carefully before review begins. Your training materials should indicate how to code all sorts of documents, to prevent reviewers from individually making different judgement calls. For instance, without explicit instruction, some reviewers may mark as “relevant” only one copy of a document with duplicates, on the assumption that duplicates in the “hot” category will be confusing for second pass reviewers or admins. However, by coding one copy as “hot” and another as “cold,” the reviewer has actually confused the system, making it more difficult for the algorithm to understand whether the document is actually important or not. Some tools, like Everlaw’s, actually do all of this cleaning for you automatically!
In addition to this initial documentation, also be sure to check in shortly after coding begins, to evaluate whether coders have interpreted the instructions the same way. Analytics that compare inter-reviewer consistency or reviewer accuracy can go a long way to diagnosing and addressing these kinds of discrepancies.
Work to reduce the bias of the testing corpus
Also important in this first pass is broad sampling of your entire data set. If you only code a limited sub-set of documents with similar characteristics, how can the model predict how this information would apply to completely different documents?
One way to do this is to create multiple training sets sampled at random from the case as a whole. Or, train your model with various related searches, not just a restricted set. For example, if you are creating a model for a custodian in a case, don’t restrict your training set to documents retrieved from searches for metadata fields with the individual’s name. Instead, consider seeking content, people, or events related to the person.
Once an initial model has been created, you can also use the coverage graph to target low-coverage areas of your document set. This can help find gaps in the algorithms knowledge base, so to speak.
Diagnose issues using the initial model’s graph
Once a predictive coding graph has been created, it can be tempting to jump right into reviewing the documents predicted to be relevant. However, you may be able to save your team time by evaluating your model’s graph first.
For example, if the majority of documents are on the right side of your distribution, the model is predicting that most of your case’s documents are relevant. While this is possible, it can also be time-consuming to review. Checking first can identify whether issues – like reviewers confused about what constitutes relevance or accidental batch-coding of documents as hot – may exist. By seeking out these kinds of issues, you can solve for them before they become a big clean-up effort.
Hopefully, these 3 tips help improve your use of predictive coding, independent of your case approach or meet-and-confer requirements. Happy (predictive) coding!