The Lawyer’s Guide to Prompt Whispering
How Legal Professionals Can Get the Most Out of Large Language Models
by Claire Spafford, Jennie Robinson, and Simone Wasbin
With the introduction of ChatGPT, the ability to type a prompt into a chat window and have it instantly return a unique, AI-generated reply has become the latest technological marvel. In a matter of seconds you can have entire pieces of content, including articles, social media posts, code, emails, lists, and more, completed without inputting any specific knowledge of your own.
While this sort of functionality is still making its way into the legal space, generative AI technology is by no means a pipe dream. Legal professionals are using it every day to manage their caseloads, conduct research, create initial drafts, review documents, and more. With EverlawAI Assistant, attorneys and organizations of all sizes are taking advantage of tools like review and writing assistance, machine translation, advanced analytics, and more to shorten time to insight and get to the truth faster.
Prompting generative AI models is one of the ways in which attorneys are discovering how to guide generative AI to produce the most valuable work product. Prompts are user-provided inputs to which large language models are meant to respond.
Structuring inputs in a way that can be understood by the model is essential to it performing your requested task.
Since these models are trained on human language, it’s important to be as detailed and explicit as possible to get the most out of the results.
Tools such as EverlawAI Assistant's Coding Suggestions, which makes use of LLM prompting, represent the next iteration of generative AI technology for legal professionals. Coding Suggestions evaluates documents and suggests codes for them to speed up the review determinations process, allowing legal teams to direct their time and resources toward more important tasks.
Understanding Coding Suggestions
Coding Suggestions allows users to evaluate documents in accordance with their coding sheet, which contains all the coding categories a user has created. For every code configured for use, EverlawAI Assistant will offer a suggestion of whether it should be applied to the document or not, and provide rationale for its suggestion based on analysis of the document text. Users can easily apply, remove, or replace a code directly in the review assistant based on the suggestion.
For example, if there’s a coding category related to the implicit suggestion of collusion by Company X, Coding Suggestions will evaluate a users’ documents and suggest which ones fit this coding criteria. It will also offer rationale for why it chose those documents, so users can evaluate the fit for themselves, and give them the option to apply, remove, or replace this code.
Users can use Coding Suggestions to speed up review determinations, or configure the tool to surface difficult-to-find, material insights from documents. It can also be used as a quality control tool for existing review work.
AI features like Coding Suggestions accelerate processes that are otherwise time-consuming. Whether you have thousands of documents or just a few, Coding Suggestions will streamline the review determinations process.
The Art of Prompting
To get the desired results from the Coding Suggestions feature, you have to understand prompting. Although you might not see it hanging in the walls of the Louvre or adorning the coveted stage of a Sotheby’s auction, prompting these models has become an art form.
Prompting large language models requires the same context and nuance you would provide when explaining a topic to someone who might be unfamiliar with it. There are three descriptions that EverlawAI Assistant uses when generating Coding Suggestions to help provide this context―the case description, coding category description, and code description.
The case description sets the stage as an overview of the case itself and the main issues at play. The coding category description then offers an opportunity to delve deeper, outlining relevant information for coding decisions to be made under a specific category and defining additional case context. The code description is where the criteria for a certain code is listed, as well as any necessary information that might not appear directly in the document text.
Here are some best practices to consider as you get started:
Case
Summarize the overall case, including the main issues and arguments at play. Define the key entities and their roles, and any other information that is important context for making coding decisions. This is a good space to provide any important information about the case that is likely relevant across multiple code categories.
Category
Include information that is relevant for coding decisions to be made for most codes under this category, but not relevant to other coding categories. When applicable, include relevant case context not already included in the case description. Define relevant terms, entities, and events.
Code
This is the space to write out the criteria indicating that this code should be applied to the document. Describe information, attributes, or qualities found in document text that would suggest the application of this specific code. Include information that is unlikely to appear directly in the text that could be important to evaluating a code.
Writing Strong Prompts for Coding Suggestions
Generative AI models are trained on human language, meaning they can understand nuance much as a normal person would. It’s important to be as straightforward as possible when prompting, and define connections between people, places, and events. Here are a few helpful tips:
Avoid Ambiguous Language and Provide Context
Be aware of language that is ambiguous or subjective. If your prompt contains words or expressions that could be open to interpretation, explicitly state your intention. Define technical terms, jargon, acronyms, and other language that might be confusing.
Ambiguous terms to avoid: Misleading, unusual, suspicious, reckless, effective, generous
Define acronyms: “CDR refers to the company’s central data repository”
Define the Scope
Depending on the amount of documents you’re hoping to capture with your search, you might use language that broadens or narrows the scope.
Broaden the scope: Language like “explicitly or implicitly related to…” or “...including similar or related information even if described in different terms”
Narrow the scope: Language like directly, specifically, exactly, only
Specify Features and Information
Specify or provide examples of the features or information you want the search results to focus on. Indicate whether the examples provided are exhaustive.
Non-exhaustive examples: “...reports on earnings, including but not limited to internal profit and loss reports, minutes from earnings calls, or SEC Form 10-k filings”
Hit the Right Tone
Describe the sentiment or tone that suggests a specific code applies in that circumstance. Also be affirmative with examples and instructions. Say “do” instead of “don’t”.
Negative language to avoid: “Do not look for internal communications between employees if Company A”
Positive language to use: “Focus only on external communications involving an employee of Company A and at least one member of another organization”
If the results you receive aren’t quite what you expected given what you know about the document details, experimenting with the wording of the additional directions may help.
A Sample Coding Suggestions Prompt For a Generative AI Model
Category: Issues, Code: Collusion
Category Prompt: These are codes capturing documents relevant to various sub-issues in the matter. For these codes, documents from and by employees of Company X and Company Y can be particularly important. Company X may also appear as ‘ABC Corp’, and Company Y was formerly known as ‘M Enterprises’.
Code Prompt: Identify documents containing language that explicitly or implicitly suggests collusion between Company X and Company Y. This includes but is not limited to communications that reference ‘strategic partnerships’, ‘coordinated efforts’, or ‘joint ventures’. In the context of this inquiry, ‘collusion’ is defined as any agreement or cooperative effort, whether formal or informal, aiming to manipulate market conditions or pricing strategies. Make sure to capture both direct communications and any indirect references or inferences made in internal memos, emails, or meeting minutes. Communications that have a tone of secrecy or concealment about these topics may be particularly interesting. Financial information indirectly evidencing collusion or business conditions can also be relevant, such as internal profit and loss reports, projection models, or pricing calculations.
How this prompt fits the criteria:
Avoid ambiguous language and provide context. The prompt clearly defines ‘collusion’ and gives specific examples of expressions that might indicate it, like ‘strategic partnerships’.
Define the scope. The prompt uses phrases like “explicitly or implicitly suggests” and “includes but is not limited to” to widen the scope.
Specify features or information. The prompt includes a section on financial information, providing specific, non-exhaustive examples of relevant documents (e.g. “internal profit and loss reports, projection models...”)
Alternate entity references. The prompt accounts for different names of the companies involved (e.g. “Company X” may also appear as “ABC Corp”).
Defining Technical Terms. The prompt defines the main term of interest, ‘collusion’, in the context of the investigation.
Affirmative examples. The prompt specifies what to focus on (e.g. “identify any documents containing language...”) rather than what to exclude.
Hit the right tone. The prompt requests to focus on documents that exhibit a tone of secrecy or concealment, linking the sentiment to the investigation's context.
The Generative AI Toolbox
Coding Suggestions is yet another tool you can use to help deliver results for your clients.
A small investment in learning these generative AI models and fine-tuning the prompts you input can pay dividends later on, especially when it comes to large projects. Leveraging generative AI to accelerate time-consuming processes like first-pass review allows you to direct resources to the most important parts of your case.
To learn more about Coding Suggestions and EverlawAI Assistant, request a demo today.
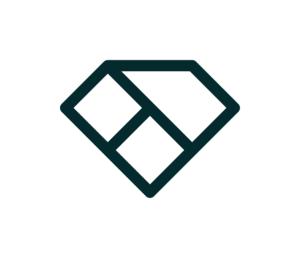
Claire, Jennie, and Simone contributed to the creation of Coding Suggestions, and help lead the responsible implementation of generative AI at Everlaw.