A Sprint to the Finish
How GenAI Can Deliver Faster, Better, Cheaper Edisclosure Document Reviews
by Ryan Hockley
Imagine the following situation: Your client has initiated a claim to prevent a competitor from unlawfully acquiring a team of key employees and going after your client’s customers and business, in breach of restrictive covenants. The court has granted an expedited trial, and there are weeks, rather than months or years, to work through the directions to trial.
First up is disclosure, the task of identifying relevant and non-privileged documents for disclosing to the other parties. After the collection, processing, deduplication and application of agreed search terms, your client has 150,000 documents to review – in three weeks. If this deadline is missed, it will impact witness statements and expert reports, and the defendants will kick up a stink; their disclosure task is minimal in comparison.
Amongst numerous issues going back several years, you’re looking for evidence of a conspiracy amongst the departing team members. It’s unlikely that they will have used complete and express sentences to discuss the move on your client’s email and messaging systems, but it is just possible that there are fragments or hints of decisions taken or offline communications that will help.
Throwing Bodies at the Problem: The Human-Only Review
The litigator’s first instinct might be to “throw bodies” at the problem and work out how to staff the review to complete within the time available. We’ll focus on the first-level review (1LR) for simplicity.
The calculation might go as follows:
Estimate the number of documents a reviewer will be able to code (this is shorthand for comprehending the content and classifying each document as relevant or not relevant and privileged or not privileged) per hour. Sixty documents per hour is not an unreasonable starting point, although this only leaves one minute per document, which might not hold if the documents are unusually long and/or complex.
Work out the number of reviewer hours required to code all the documents – 150,000 documents at 60 docs per hour would require 2,500 review hours.
Calculate the reviewer team size that would be necessary to complete 1LR within three weeks, assuming 35-hour working weeks. With 2,500 hours of review, across three weeks, with reviewers working 35 hours per week, 24 reviewers would be needed.
An estimate of the cost would be to multiply 2,500 hours by the reviewers’ hourly rate – let’s assume £60 per hour (it keeps the maths easy!), which would equate to £150,000. This amount does not include the considerable overheads and costs to assemble and manage a team of 24 people.
The high-level numbers rule this approach out almost immediately. It’s impractical and expensive, and it only just completes 1LR by the deadline, with limited headroom (both in budget and timetable) for second-level review (2LR) or other quality control tasks.
There’s also a significant qualitative concern associated with getting 24 reviewers new to the matter up to speed and making consistent coding decisions at a rate of a minute per document. Contrary to popular belief (in some quarters), the 100% human approach is far from the “gold standard” in terms of accuracy.
Speeding Up Review with TAR
It is somewhat unrealistic to think that anyone would advise a human-only approach for this review in 2025. Predictive Coding, or technology assisted review (TAR) is well-established, and consideration of its use is expected in most cases.
TAR algorithms look for patterns in documents which have been coded relevant by human reviewers, and a model is created to predict the relevance of the remaining documents in the pool. When TAR works, it continuously promotes relevant documents to the top of the review queue for the human review team to work through. Over time, the relevance rate will fall, hopefully reaching a point where it is not proportionate to continue the human review.
At this point, a statistically significant sample of the remaining, unreviewed documents is analysed to see if any relevant documents were not identified in earlier review – called an “elusion test”. If the test is passed, the review stops at that point and human review is avoided for a proportion of documents.
Contrary to popular belief (in some quarters), the 100% human approach is far from the “gold standard” in terms of accuracy.
We can say with some confidence that it will be faster and cheaper than pure human review, but what does this mean in cold, hard numbers? The unsatisfactory answer is “it depends”. Amongst other variables, it depends on whether the algorithm can create an effective model to differentiate between relevant and non-relevant documents and on the relevance rate in the data set. In the scenario where you only have three weeks to review 150,000 documents, you would most likely have to take a conservative approach to resourcing to be confident of completing in time. You could still be looking at a team of 10 to 15 reviewers, with associated management costs, leading to a total cost of about half the human-review price.
From a quality point of view, TAR itself will not improve the quality of human decisions, but it should create some headroom for 2LR and quality control. Research shows that TAR can outperform human reviewers. However, TAR doesn’t “understand” the documents or the issues in play. There could be relevant documents which do not match the TAR model and, as such, would be predicted “non-relevant”. If they are not picked up in the elusion test, they will be missed. These could include smoking gun documents for the conspiracy case which do not have features in common with documents relevant to other issues.
GenAI Saves the Day – and the Dollars
We now have the option of using sophisticated large language models’ generative AI functionality (GenA) to review documents and make coding decisions. Unlike the pattern modelling of TAR, GenAI makes coding decisions about documents based on a (simulated) understanding of their content combined with a case overview and coding criteria.
GenAI coding costs will vary between platforms and other features, including the average length of documents under review. For the purposes of this analysis, let’s assume an average rate of £0.30 per document – likely to be at the upper end of the range. Therefore, the cost for a 1LR coding decision for every document in our scenario will be £45,000. We’ll need to factor in project management costs to configure, test and iterate upon the GenAI instructions, but these should lower (perhaps considerably so) than the costs of overseeing review teams of 10 to 24 people.
The duration of review will depend on the approach taken. It is recommended to run through a few iterations of testing the configuration over a sample of documents and checking the results before running it against the whole data set. Often, discrepancies between GenAI coding decisions and the litigator’s expectations will be due to ambiguities or gaps in the GenAI coding configuration, which can be refined between iterations. It should only take a day or so to settle the configuration and then a matter of hours to run it against the rest of the data set.
Because the entire process can be completed in a matter of days, rather than weeks, the case team can devote itself more fully to the merits – rather than disclosure.
The GenAI approach would be cheaper and faster than human review, but how would it compare in terms of quality and accuracy? We’re certainly not at the stage where complete reliance on GenAI coding decisions without appropriate human oversight and quality measures would be recommended. GenAI should be treated like a bright paralegal or freelance reviewer (or a reviewer of any level reviewing hundreds of documents a day): unlikely to get 100% of decisions right. Just as one should implement appropriate quality workflows for our human review team, one should be prepared to do just the same for the GenAI decisions.
However, GenAI coding functionality (at least as it has been implemented in the Everlaw platform) has three distinct advantages over its human rivals:
For every single document reviewed, it records the phrases and sections underpinning its determination on relevance. Try getting this information out of the heads of reviewers at the end of a 35-plus hour week of review!
GenAI makes a determination based on the whole document, every time. That may not be the case for our human review team trying to review hundreds of documents a day at a rate of a document a minute.
GenAI explains itself. In addition to citing supporting evidence in the documents, Everlaw’s Coding Suggestions provide a rationale for why the suggested code is given, creating a detailed record of how the coding determinations were made – far more detailed than in other review approaches.
If the planned workflow involves the advising law firm associates reviewing all 1LR documents coded relevant in a second-level review (a common approach), then the quality control workflow needs to focus on the pool of documents which have been determined by GenAI to be not relevant.
If this sounds familiar, it’s because it is entirely analogous to the TAR approach: a requirement to sample the documents which will not be human reviewed. The elusion test will have a role to play, but GenAI functionality (at least how it has been implemented by Everlaw) allows us to run a couple of more-targeted QC reviews:
Coding decisions are not entirely binary. Everlaw classifies a match for a particular relevance code as one of four options, depending on the confidence of its decision: Yes, Soft Yes, Soft No, No. QC review effort can be targeted towards the documents that GenAI is less certain about how to categorise.
Multiple Coding Suggestions can be made at a time. This allows for issue-specific codes with a tighter description of each issue or for different ways of constructing prompts for the same issue – for example, by including both narrow and broad definitions. Going back to our search for documents evidencing conspiracy or offline comms, we could add issue codes to search for concepts indicating concealment, offline communications, or general wrongdoing, irrespective of how closely such documents resemble other issues in the matter.
The time and budgetary headroom created by GenAI functionality creates an opportunity for quality control measures to be targeted and planned and then performed by associates familiar with the issues in the matter. And because the entire process can be completed in a matter of days, rather than weeks, the case team can devote itself more fully to the merits – rather than disclosure.
Winning the Race
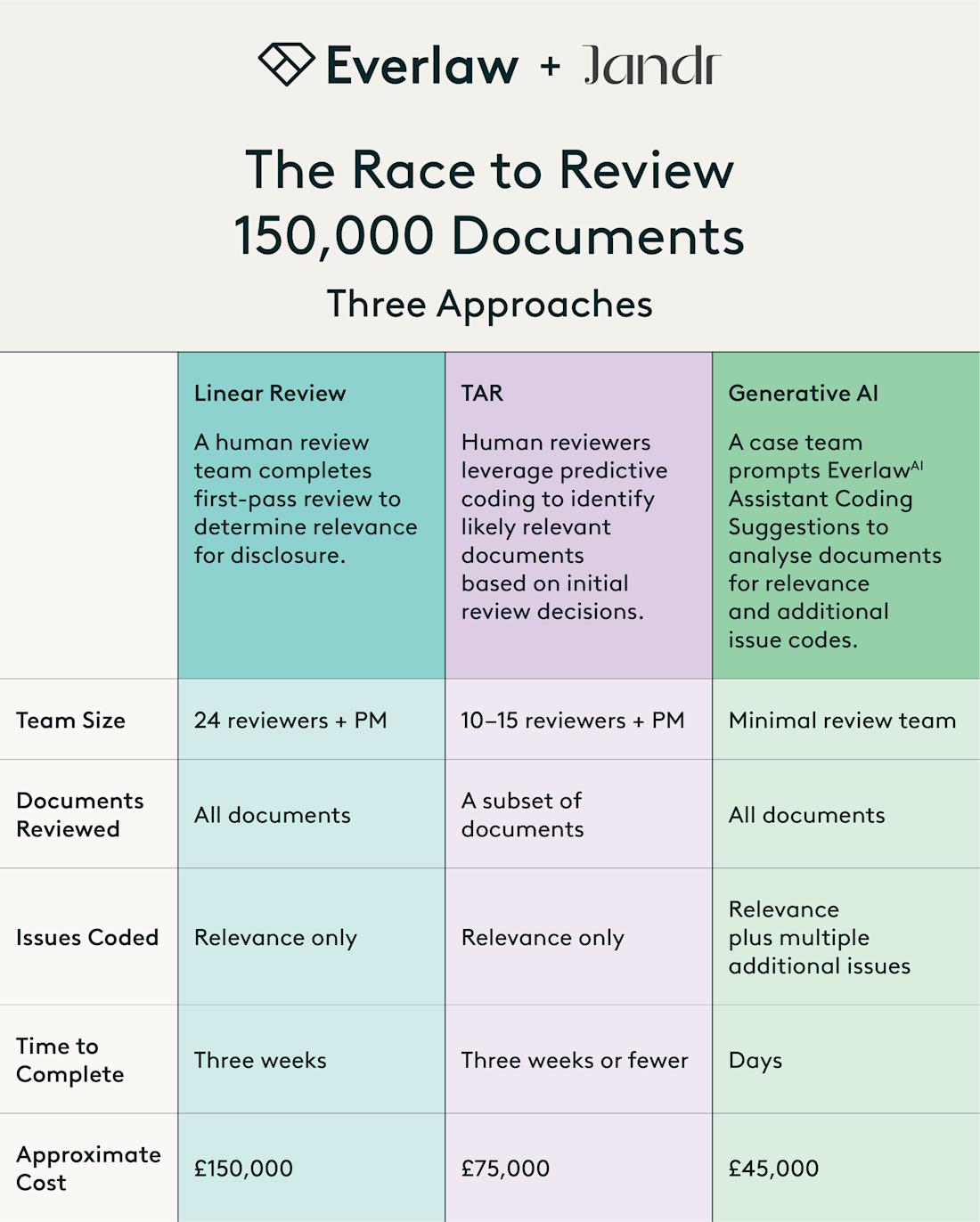
In practice, there’s a role for all three approaches in combination, in proportions which depend on the specifics of each review. Human review will always have a role to play, albeit that GenAI should result in a shift towards smaller teams of more experienced reviewers. TAR can work alongside GenAI, developing its model from human and GenAI decisions to prioritise predicted relevant documents for GenAI coding and potentially avoiding GenAI coding costs for the least relevant documents.
We believe that the prospect of GenAI-powered reviews, justified with the established elusion test, can realistically deliver faster, cheaper and better edisclosure document review outcomes.
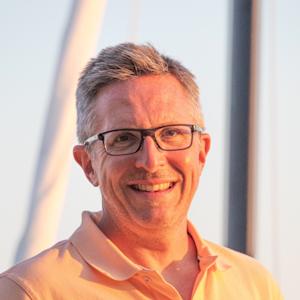
Ryan Hockley is a commercial and disputes lawyer and the founder and director of the alternative legal services provider Jandr. Jandr Legal provides experienced legal freelancers, technology consulting and project management expertise on legal projects such as document reviews in edisclosure, investigations and DSARs, due diligence exercises and bulk contract production/review/amendment tasks.